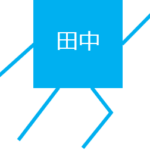
TensorFlowを使ってみたくて、公式にあったチュートリアルを実行してみるよ!
概要
TensorFlowの公式サイトにMNIST の学習をするチュートリアルがあったので、備忘録を残しておきます。
実行環境
・TensorFlow 2.0
・Python 3.7
・centOS
コード
#!/usr/local/bin/python3
import tensorflow as tf
from tensorflow.keras.layers import Dense, Flatten, Conv2D
from tensorflow.keras import Model
# Read mnist datasets
# x_<...> is [number x y]
# tuple of numpy.ndarray.
mnist = tf.keras.datasets.mnist
(x_train, y_train), (x_test, y_test) = mnist.load_data()
# Cast unsigned char to float(<=1).
x_train, x_test = x_train / 255.0, x_test / 255.0
# Add a channels dimension.
# tf.newaxis is None
# x_<> is [number x y channel]
x_train = x_train[..., tf.newaxis]
x_test = x_test[..., tf.newaxis]
# Convert ndarray to tf.data.Datasets object.
train_ds = tf.data.Dataset.from_tensor_slices(
(x_train, y_train)
)
test_ds = tf.data.Dataset.from_tensor_slices(
(x_test, y_test)
)
# Add shuffle buffer_size to tf.data.Dataset object,
# and batch size.
train_ds = train_ds.shuffle(10000).batch(32)
test_ds = test_ds.batch(32)
# Create model using model subclassing API in Keras.
# Model is constructure of NN.
class MyModel(Model):
def __init__(self):
super(MyModel, self).__init__()
self.conv1 = Conv2D(32, 3, activation='relu')
self.flatten = Flatten()
self.d1 = Dense(128, activation='relu')
self.d2 = Dense(10, activation='softmax')
def call(self, x):
x = self.conv1(x)
x = self.flatten(x)
x = self.d1(x)
return self.d2(x)
model = MyModel()
# Select loss function
loss_object = tf.keras.losses.SparseCategoricalCrossentropy()
# Select optimizer
optimizer = tf.keras.optimizers.Adam()
# Select metrics for measurements
train_loss = tf.keras.metrics.Mean(name='train_loss')
train_accuracy = tf.keras.metrics.SparseCategoricalAccuracy(
name='train_accuracy'
)
test_loss = tf.keras.metrics.Mean(name='test_loss')
test_accuracy = tf.keras.metrics.SparseCategoricalAccuracy(
name='test_accuracy'
)
@tf.function
def train_step(images, labels):
# Recode formula by tape
with tf.GradientTape() as tape:
predictions = model(images)
loss = loss_object(labels, predictions)
# Calculate gradient
# model.trainable_variables give list of weight
gradients = tape.gradient(loss, model.trainable_variables)
# Update weight value
optimizer.apply_gradients(
zip(gradients, model.trainable_variables)
)
train_loss(loss)
train_accuracy(labels, predictions)
@tf.function
def test_step(images, labels):
predictions = model(images)
t_loss = loss_object(labels, predictions)
test_loss(t_loss)
test_accuracy(labels, predictions)
# Set EPOCHS number.
EPOCHS = 5
for epoch in range(EPOCHS):
for images, labels in train_ds:
train_step(images, labels)
for test_images, test_labels in test_ds:
test_step(test_images, test_labels)
template = 'Epoch {}, Loss: {}, Accuracy: {}, Test Loss: {}, Test Accuracy: {}'
print (
template.format(
epoch+1,
train_loss.result(),
train_accuracy.result()*100,
test_loss.result(),
test_accuracy.result()*100
)
)
train_loss.reset_states()
train_accuracy.reset_states()
test_loss.reset_states()
test_accuracy.reset_states()
解説
import tensorflow as tf
from tensorflow.keras.layers import Dense, Flatten, Conv2D
from tensorflow.keras import Model
必要なモジュールをインポートします。tensorflow.keras.layers の下に、ニューラルネットワークで使う層が大体入っています。
# Read mnist datasets
# x_<...> is [number x y]
# tuple of numpy.ndarray.
mnist = tf.keras.datasets.mnist
(x_train, y_train), (x_test, y_test) = mnist.load_data()
mnist = tf.keras.datasets.mnist
(x_train, y_train), (x_test, y_test) = mnist.load_data()
上記でMnist のデータセットをダウンロードしてきてくれます。そして、x_train, y_train, x_test, y_test にデータが入ります。x_train が入力データで、よく見るMNIST の画像データが入っています。y_train が正解ラベルで、0~9の数字が入っています。x_test, y_test はテスト用のデータで、x_train, y_train と構造は一緒です。ちなみに、numpy配列です。
# Cast unsigned char to float(<=1).
x_train, x_test = x_train / 255.0, x_test / 255.0
取得した画像データは255 ~ 0 の1byte型(unsigned int? unsigned char?)です。しかし、Tensorflow で使える型はfloat型なので、型を変換する必要があります。なので、255.0 で割って、 1 ~ 0 に変換して、float型にしています。
# Add a channels dimension.
# tf.newaxis is None
# x_<> is [number x y channel]
x_train = x_train[..., tf.newaxis]
x_test = x_test[..., tf.newaxis]
Tensorflow のニューラルネットワークを通すためにチャネルを追加する必要があるので追加しています。tf.newaxis をprint すると Noneが返ってきます。
# Convert ndarray to tf.data.Datasets object.
train_ds = tf.data.Dataset.from_tensor_slices(
(x_train, y_train)
)
test_ds = tf.data.Dataset.from_tensor_slices(
(x_test, y_test)
)
# Add shuffle buffer_size to tf.data.Dataset object,
# and batch size.
train_ds = train_ds.shuffle(10000).batch(32)
test_ds = test_ds.batch(32)
x_train, y_train を組み合わせて、dataset型(俗称)に変換します。dataset型 にすることで、入力データを正解ラベルの組み合わせを崩さずにランダムに選別したりできます。.shffle()で何枚分をランダムに選択するかを指定します。.batchでバッチ数を指定します。
# Create model using model subclassing API in Keras.
# Model is constructure of NN.
class MyModel(Model):
def __init__(self):
super(MyModel, self).__init__()
self.conv1 = Conv2D(32, 3, activation='relu')
self.flatten = Flatten()
self.d1 = Dense(128, activation='relu')
self.d2 = Dense(10, activation='softmax')
def call(self, x):
x = self.conv1(x)
x = self.flatten(x)
x = self.d1(x)
return self.d2(x)
model = MyModel()
tf.keras.Model を継承し、ニューラルネットワークを作成します。ネットワークだけを変えるなら、ここを変えるだけで良いです。
# Select loss function
loss_object = tf.keras.losses.SparseCategoricalCrossentropy()
# Select optimizer
optimizer = tf.keras.optimizers.Adam()
# Select metrics for measurements
train_loss = tf.keras.metrics.Mean(name='train_loss')
train_accuracy = tf.keras.metrics.SparseCategoricalAccuracy(
name='train_accuracy'
)
test_loss = tf.keras.metrics.Mean(name='test_loss')
test_accuracy = tf.keras.metrics.SparseCategoricalAccuracy(
name='test_accuracy'
)
損失関数と重みの更新方法(オプティマイザ)を指定します。
@tf.function
def train_step(images, labels):
# Recode formula by tape
with tf.GradientTape() as tape:
predictions = model(images)
loss = loss_object(labels, predictions)
# Calculate gradient
# model.trainable_variables give list of weight
gradients = tape.gradient(loss, model.trainable_variables)
# Update weight value
optimizer.apply_gradients(
zip(gradients, model.trainable_variables)
)
train_loss(loss)
train_accuracy(labels, predictions)
@tf.function
def test_step(images, labels):
predictions = model(images)
t_loss = loss_object(labels, predictions)
test_loss(t_loss)
test_accuracy(labels, predictions)
@tf.functionはイタレータでこれをコメントアウトするとTensorflow 2.x 動作、そのままでTensorflow 1.x 動作をします。2.x は途中計算を見ることができる代わりに低速、1.x 系は途中計算を見れない代わりに高速で動くという特徴があります。
# Set EPOCHS number.
EPOCHS = 5
for epoch in range(EPOCHS):
for images, labels in train_ds:
train_step(images, labels)
for test_images, test_labels in test_ds:
test_step(test_images, test_labels)
template = 'Epoch {}, Loss: {}, Accuracy: {}, Test Loss: {}, Test Accuracy: {}'
print (
template.format(
epoch+1,
train_loss.result(),
train_accuracy.result()*100,
test_loss.result(),
test_accuracy.result()*100
)
)
train_loss.reset_states()
train_accuracy.reset_states()
test_loss.reset_states()
test_accuracy.reset_states()
EPOCH 数だけ学習と途中結果の出力をします。 .reset_states() をしないと、値が加算されていって、正解率が100% を超えます。気を付けてください。
蛇足
Tensorflow の公式サイトのドキュメントは初心者には難しかったです。
コメント